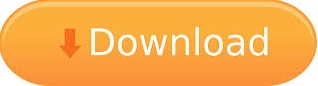
However, these studies focused on ATE and there are still no accessible demonstrations of g-computation applied to ATT and ATU. To date, there are several didactic demonstrations for g-computation and applied examples for projecting the impact of hypothetical interventions aimed at reducing risk factors for coronary heart diseases or diabetes in adult populations, or at reducing early childhood adiposity. small standard errors) and more stable estimates in parametric settings and can better handle heterogeneity involving time-varying exposure and confounding. G-computation is often seen as a viable alternative to IPTW because g-computation produces more efficient (i.e. The parameters of a MSM can be consistently estimated using two classes of estimators: the g-computation algorithm and the inverse-probability of treatment weighting (IPTW). Among these, the marginal structural models (MSMs) were designed to estimate marginal quantities (i.e., not conditional on other covariates). Robins introduced the “g-methods” to estimate such quantities using observational data. under perfect randomization with perfect compliance or when there is no unmeasured confounders) and there is no effect measure modification by the covariates. All three quantities will be equal when the covariate distribution is the same among the treated and the untreated (e.g. Interestingly, the ATE can be seen as a weighted average of the ATT and the ATU. This latter quantity is referred to as the average treatment effect on the untreated (ATU). For instance, one might wish to project the effect of the smoking cessation intervention in a city that did not receive the intervention in order to gauge its potential impact when such intervention is actually implemented. ATT) but not that on those among whom the intervention was never intended.Īlternatively, researchers may be interested in estimating the potential impact of an existing program in a new target (sub-) population. ATE), researchers and policymakers might be interested in explicitly evaluating the effect of the intervention on those who actually received the intervention (i.e. Although the overarching goal of such evaluation may be to assess the impact of such intervention in reducing the prevalence of smoking in the general population (i.e. For instance, consider a scenario where a government has implemented a smoking cessation campaign intervention to decrease the smoking prevalence in a city and now wishes to evaluate the impact of such intervention. However, in economics and evaluation studies, it has been noted that the average treatment effect among units who actually receive the treatment or intervention (average treatment effects on the treated, ATT) may be the implicit quantity sought and the most relevant to policy makers. This quantity provides the average difference in outcome between units assigned to the treatment and units assigned to the placebo (control). In epidemiology, (bio)statistics and related fields, researchers are often interested in the average treatment effect in the total population (average treatment effect, ATE). Its use should be encouraged in modern epidemiologic teaching and practice. The g-computation algorithm is a powerful way of estimating standardized estimates like the ATT and ATU. In our illustrative example, the effect (risk difference ) of a higher education on angina among the participants who indeed have at least a high school education (ATT) was −0.019 (95% CI: −0.040, −0.007) and that among those who have less than a high school education in India (ATU) was −0.012 (95% CI: −0.036, 0.010). The estimates for ATT, ATU and average treatment effect (ATE) were of similar magnitude, with ATE being in between ATT and ATU as expected. To obtain marginal effect estimates for ATT and ATU we used a three-step approach: fitting a model for the outcome, generating potential outcome variables for ATT and ATU separately, and regressing each potential outcome variable on treatment intervention. In this paper we illustrate the steps for estimating ATT and ATU using g-computation implemented via Monte Carlo simulation. Average treatment effects on the treated (ATT) and the untreated (ATU) are useful when there is interest in: the evaluation of the effects of treatments or interventions on those who received them, the presence of treatment heterogeneity, or the projection of potential outcomes in a target (sub-) population.
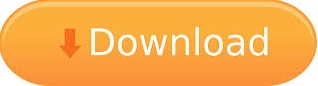